For rigorous training in statistical and machine learning methodology for data science, we recommend the Statistics and Data Science B.S., offered by the PSTAT department. But data science is cross-disciplinary by nature, and students outside of PSTAT can also draw from a number of other classes to broaden their potential for research and post-graduate employment. We provide a sampling of UCSB courses featuring data science training (and programming) below.
Core Courses for an Interdisciplinary Data Science Curriculum
Introduction to Data Science 1 (aka DS 1)
CMPSC 5A
This course introduces students to inferential thinking and computational thinking in the context of real-world problems. How does one analyze data resulting from a real-world process in order to understand the process? The course teaches critical concepts and skills in computer programming and statistical inference, in conjunction with hands-on analysis of real-world datasets, including economic data, document collections, geographical data, and social networks. It delves into social and legal issues surrounding data analysis, including issues of privacy and data ownership.
CMPSC 5A targets students outside of CS and PSTAT departments and has no prerequisite requirements. Students interested in data science might consult with their academic advisors about the potential for replacing PSTAT 5A with the CMPSC 5A/5B sequence, which explores the subject matter in greater detail over two quarters.
Although CMPSC 5A is similar in structure to CMPSC 8, this course does not replace the CMPSC 8 requirement for the Data Science and Statistics major in PSTAT.
Introduction to Data Science 2 (aka DS 2)
CMPSC 5B
This course continues the themes of Data Science 1. Students explore the data science lifecycle, including question formulation, data collection and cleaning, exploratory data analysis and visualization, statistical inference and prediction, and decision-making. The course focus is on languages for transforming and analyzing data; machine learning methods including regression, classification and clustering; principles behind data visualizations; concepts of measurement error and prediction; and techniques for scalable data processing.
Prerequisite: CMPSC 5A.
Principles & Techniques of Data Science (aka DS 3)
PSTAT 100
This course explores the data science lifecycle, including question formulation, data collection and cleaning, exploratory data analysis and visualization, statistical inference and prediction, and decision-making. It focuses on quantitative critical thinking and the key principles and techniques that are needed. These include languages for transforming, querying and analyzing data; algorithms for machine learning methods including regression, classification and clustering; principles behind creating informative data visualizations; statistical concepts of measurement error and prediction; and techniques for scalable data processing.
Pre-requisite is PSTAT 120A, Math 4A, and prior experience with Python or another programming language.
Data Science Capstone
Fall: CMPSC 190DD / PSTAT 197A
Winter: CMPSC 190DE / PSTAT 197B
Spring: CMPSC 190DF / PSTAT 197C
In the first quarter of this three-course sequence, students will study data science from the systems engineering perspective, introduce and address a variety of ethical issues that arise in data science projects, and engage students in project-based learning through a series of carefully selected and curated data science studies. A major overarching goal is to prepare students to make a positive impact on the world with data-intensive methodologies. In line with this, we will study fairness, ethics, and responsible data practice. Another major focus will be on correctly interpreting, explaining, and communicating the results of analyses. This component of the course will focus on decision making under uncertainty, the role of correlation and causation, and drawing attention to common statistical traps and paradoxes that drive erroneous conclusions.
Prerequisites: Students should have a background in computing and statistics before enrolling in capstone.
Suggested courses include the following:
PSTAT students: 120A, 100, 126, 131
COMSC: 16, 130A, 165A, 165B
In the second and third quarters, students form teams and collaborate with industry partners and research labs. Current course and project information is here.
Select Courses for Domain Knowledge
Some of the courses listed below have major restrictions and prerequisites. Refer to home departments and the Registrar for confirmation of prerequisites and descriptions. Students in the PSTAT Statistics and Data Science major should contact PSTAT advisors before enrolling in any non-PSTAT courses.
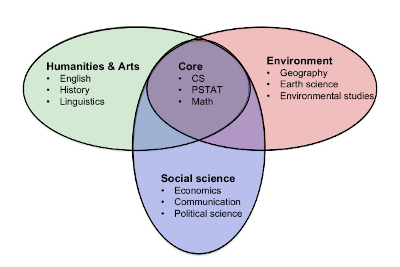
Anthropology
Data Analysis for the Social Sciences with R
ANTH 123MG
An introduction to the scientific process and data analysis using the programming language R. Students harness fundamentals of data manipulation, visualization, and research workflows to refine and present hypotheses and results on social science datasets. Introduces modeling methods.
Quantitative Data Analysis in Archaeology
ANTH 182
This course is an introduction to the practical analysis of commonly encountered archaeological data using simple quantitative and statistical procedures such as exploratory data analysis, sampling, regression, and spatial analysis. The course is taught in a computer-assisted (mutimedia) format.
Art
Introduction to Computer Programming and the Arts
ART 22
Using a project-based approach, the basic components of web development and computer programming are explored in different markup and programming languages such as HTML/CSS, JavaScript, and Processing. The class is intended to create a general understanding of computer programming, its use and cultural implications, as well as provide a foundation for utilizing programming in a wide range of projects, from traditional to new media.
Biology
Programming in Biology
MCDB 170
Studying complex biological systems can be greatly facilitated by modern computing technologies. This course introduces essential computer programming concepts and algorithms to biology major students. Students learn logics of programming and apply it to gene sequence analysis (bioinformatics), simulation of dynamic systems (systems biology), and data analysis (statistics in biology).
Prerequisites: MCDB 1A, EEMB 2 and MCDB 1B; and MCDB 101A or EEMB 129; and PSTAT 5A or PSTAT 5LS or MATH 4A.
Biological Dynamics
MCDB 172
An introduction to mathematical models and computer simulations used to describe and understand time varying biological systems. Learning objectives: Survey mathematical methods for describing the dependence on time of biological phenomena. Illustrate how to construct mathematical models to gain insights into complex biological systems. Develop working knowledge of a python code base that enables future evaluation of common classes of models applied to the study of biological dynamics.
Prerequisites: MCDB 1A, EEMB 2 and MCDB 1B; and MCDB 101A or EEMB 129; and either MATH 4A or MCDB 108C.
Biochemistry - Computational & Systems Biology
MCDB 108C
Models of Biochemical and Cellular Systems. Introductory systems-biology approach to model the design and the function of biological systems. Students will develop an intuition about physical concepts that are fundamental to discuss how biological organisms acquire and process information from the environment. Those concepts and tools will cover probabilities and basic dynamical systems theory. Students will build models of processes of increasing complexity, ranging from viral dynamics, bacterial resistance to drugs, the maintenance of homeostatic equilibrium (trp operon), biological oscillators (mitotic clock) and genetic switches underlying cellular decisions (bacteriophage lambda and lac operon).
Prerequisite: MCDB 108A-B; Physics 6A-B-C; Math 34A-B or Math 3A-B or Math 2A-B.
Communication
Statistical Analysis for Communication
COMM 87
An introduction to basic statistical concepts and applications in communication research. Through lecture and computer labs, students are exposed to the principles and procedures involved in quantitative data analysis.
Data Science in Communication: Python and Reproducible Research
COMM 160DS
This 10-week course introduces senior undergraduates in the Department of Communication to the fundamentals of data science using Python, focusing on reproducible research practices. Students will learn essential Python libraries, data visualization techniques, and principles of reproducible research to analyze and interpret data in the context of communication and social science research. By the end of the course, students will be able to apply these skills to a final project analyzing real-world communication data while ensuring their work is transparent, shareable, and reproducible. Spring 2023 Syllabus
Data Science in Communication
COMM 187
Explore Data Science in Communication (and other related Social Sciences) and delve into issues of not just computational methodologies and processes (and related concepts such as "Big Data", "Machine Learning", and "Artificial Intelligence"), but also important aspects of ethics, social impact of technology, and data visualization. Understand different aspects and nuances of this topic by examining multiple case studies of the use of Data Science techniques in Communication research.
Qualitative Methods in Communication
COMM 280
This course provides an overview of qualitative research methods, including in-depth interviewing, participant observation, and grounded theory. Using exemplars, it also examines approaches to data analysis, specifically, constant comparison method, narrative and discourse analyses, and computer assisted data management.
Comparative Literature
Digital Humanities Practice
CLIT 152
In the 21st century, scholars have increasingly turned to computational methods for the analysis of large corpora of art and literature. While early methods of "distant reading" and "distant viewing" could be realized with off-the-shelf software, contemporary research in the digital humanities requires technical skills beyond ready-made tools. This course provides an introduction to computer programming for the humanities, including, but not limited to concepts from natural language processing, computer vision, and machine learning. Same course as GER 152.
Critical Artificial Intelligence
CLIT 155
Artificial intelligence now affects nearly all aspects of human life and knowledge production, from labor to language, and from fundamental physics to the arts. The pivotal role of the humanities lies in the critical analysis of the specific cultural techniques emerging from this technical revolution: new methods of language processing, image production, scientific reasoning, and social control require new critical and historical approaches. This course provides an introduction to the history and theory of artificial intelligence from the perspective of the humanities. Participants will acquire the skills to analyze and understand the design and construction of machine learning systems, and their philosophical and political implications. Same course as GER 155.
Computer Science
Introduction to Computer Science
CMPSC 8
Introduction to computer program development for students with little to no programming experience. Basic programming concepts, variables, and expressions, data and control structures, algorithms, debugging, program design, and documentation.
CMPSC 8 is a popular course for learning to program in Python, and there is often a waiting list. Students unable to enroll, and who do not necessarily require CMPSC 8 for their major, might consider CMPSC 5A as an alternative.
Intermediate Python
CMPSC 9
Intermediate topics in Computer Science using the Python programming language. Topics include object oriented programming, runtime analysis, data structures, and software testing methodologies.
CMPSC 9 is typically reserved for PSTAT majors, but interested students who have fulfilled the prerequisites can inquire about enrollment codes.
Prerequisite: CS 8 or Eng 3.
Introduction to Computational Science
CMPSC 111
Introduction to the numerical algorithms that form the foundations of data science, machine learning, and computational science and engineering. Matrix computation, linear equation systems, eigenvalue and singular value decompositions, numerical optimization. The informed use of mathematical software environments and libraries, such as python/numpy/scipy.
Prerequisite: Mathematics 4B with a grade of C or better; Mathematics 6A with a grade of C or better; Computer Science 24 with a grade of C or better.
Ecology, Evolution, and Marine Biology
Biometry
EEMB 146
Linear models and least squares fitting: simple and multiple linear regression; analysis of variance (fixed, random and mixed models; crossed and nested effects; balanced and unbalanced designs); analysis of covariance, factorial designs; incomplete layouts; use of transformations.
MATH 2A-B or 3A-B or 34A-B; and PSTAT 5A or PSTAT 5LS or PSTAT 109 or Math 4A or PSB 5.
Ecological Modeling
EEMB 179
An introduction to mathematical and computer models in studies of the natural environment with emphasis on population dynamics and species interactions. Case studies of interacting physical, chemical and biological phenomena.
MATH 34A-B or 3A-B or 2A-B.
Quantitative Methods in Biology
EEMB 247
A review of quantitative methods required to develop models of biological and ecological systems. Topics illustrated through computer exercises.
Economics
Statistics for Economics
ECON 5
An introduction to probabilistic modeling and statistical inference applied to the analysis of economic data for students with basic knowledge of calculus. Topics covered include: probability, discrete and continuous random variables, probability distributions, mean, variance, correlation, sampling, parameter estimation, unbiasedness and efficiency, confidence intervals, hypothesis testing. Computing labs with Excel.
Introduction to Econometrics
ECON 140A-B
140A - Estimation and hypothesis testing in classical linear regression models as well as violations of each classical assumption. Discrete dependent variable models and systems of simultaneous equation are also covered.
140B - Topics in econometrics including regression specification, time series econometrics, panel data, and instrumental variables.
Data Wrangling for Economics
Using R, students develop skills in organizing economic data, learning how to summarize and display data to answer substantive economic questions. Emphasis is placed on communication of results.
Electrical & Computer Engineering
Introduction to Electrical Engineering
ECE 3
Introduction to fundamental design problems in Electrical Engineering through programming in Python. This course is open to EE majors only, but listed here for comparison to Datascience 1.
Introduction to Deep Learning
ECE 180
Introduction to multilayered neural networks, early models of perceptrons and associative memory; back-propagation learning; convolutional neural networks; recurrent neural networks; attention models; application to natural language processing and computer vision. Prerequisite: open to EE, Computer Engineering, and Computer Science with upper-division standing.
English
Introduction to Digital Humanities
This course introduces important types and methods of the digital humanities (“DH”). Topics include the emergence of digital humanities as a field, “distant reading,” text encoding , text analysis (including various methods of quantitative analysis, topic modeling, and “word embedding”), artificial-intelligence “large language models,” social network analysis, and GIS mapping. A key aspect of the course is the balance it seeks between ideas and technology. Far-reaching ideas about literature and other areas of social and cultural life are reexamined from a technological perspective, and, reciprocally, current technology is thought about in relation to far older and more extensive domains of technê (human arts and skills).
Data Stories: Theory and Practice of Data-driven Narratives in the Digital Age
“Data Stories” introduces students to an increasingly important genre of discourse in today’s society: data-driven narrative--e.g., as it appears in journalism; science, medical, and political reporting; business or government writing; and even some literary and artistic forms. The course brings humanities approaches such as narrative theory, genre theory, and media theory into conjunction with readings about data journalism and data visualization to ask this central question: how do you make a good story out of data? Among other assignments, students will create a project in which they take a dataset and make a narrative about it.
Critical engagement with AI/machine learning
This seminar will work towards settling on a set of research questions about an inherently unsettled technological domain: AI/machine learning. Articulating and exploring research questions will not however exclude the practical and in fact much of the work we engage will either emerge from practice or be grounded in empirical inquiry. To attempt something like comprehensiveness for this field is implausible and indeed unfeasible, but we should be able to arrive collectively at the point of something like foundational understanding, with a clear sense of directions for future research and teaching. Although we will not proceed in this order, we will take an end-to-end approach, from data, through model architectures and the production of prediction, and ending with applications and implementations.
The Humanities and Data Science
This course explores today’s quickening mutation of the “liberal arts” into “data science,” a new universal mode of knowledge touching all fields. The course focuses on the join, but also split, between how the humanities and data science find meaning (scientific, epistemological, sociopolitical, and cultural) in patterns. Topics to be probed include: the history and present state of the humanities, the concept of “data science” (including the shape of today’s new programs and majors in the field), the idea and structures of “data,” the idea and infrastructures of “big data,” humanities corpora and datasets (including the social and ethical problem of “representative” datasets), narrativizing data, visualizing data, and interpreting data. The course includes but is not limited to approaches related to the digital humanities.
Digital Humanities: Introduction to the Field
This course provides a graduate-level introduction to the digital humanities. The course introduces major types of digital humanities work and central topics and controversies. It asks students to develop project ideas and public visibility in their intended professional field in its relation to the digital humanities. Major topics include: the emergence of the digital humanities; the relation of DH to the humanities and data science; the logic of text encoding and methods of text analysis (including quantitative analysis, topic modeling, and social network analysis); theory and issues of the archive in the digital age (including in relation to issues of diversity); space and time in the digital humanities (including mapping and timelines); data narratives; and the new horizon of neural-network artificial intelligence methods.
Environmental Studies
Quantitative Thinking in Environmental Studies
ENVS 25
Improve students' ability to deal with quantitative aspects of environmental topics by developing skills in algebra, computer use (Excel), graphing, and processing and conceptualizing environmental data by using numerical modeling. Collaborative learning is emphasized.
Geography
Principles of Environmental Data Analysis
GEOG 136
This course will provide an introduction to the principles of environmental physics and their application to ecological sciences, with a focus on programming and data analysis in Python. Course activities will use data analysis to quantify environmental patterns and processes. Emphasis will be placed developing coding skills in Python and applying these skills to environmental and biophysical problems. Course goals:
- To develop expertise in the Python programming language and the use of Python’s data science stack to effectively store, manipulate, and gain insight into environmental data.
- To be able to apply this understanding to characterize data on environmental patterns and processes at varying spatial and temporal scales.
- To use data to model environmental processes of energy and mass transfer.
Analysis and Modeling of Movement
GEOG 186
Many geographic, social, and natural systems involve dynamic processes or movement of individuals in space and time. Examples include animal migration, human mobility, disease diffusion, and natural disasters like hurricanes and wildfires. Movement is key to understanding these dynamic processes. This course reviews computational methods for analysis, modeling and simulation of movement in ecological and human systems. Students will gain an understanding of spatiotemporal processes and patterns. Students will develop computational skills to process trajectory data, analyze movement patterns, and apply movement models.
Prerequisites: students are expected to have a basic knowledge of GIS, spatial analysis, and statistical analysis, and have some programming experience with Python or R.
History
Science and the Modern World
HIST 20
Explores how science, technology and/or medicine have helped shape modern societies (roughly, 1850 - present). Themes include formation of scientic and technical communities, the interactions of science with political and pupular sculture, and the social context of knowlege production.
Linguistics
Programming for Linguists
LING 102 - Fall 2020 Syllabus
Hands-on introduction to programming in Python, with emphasis on methods for retrieving, structuring, and interacting with textual data. Focus on developing practical programming skills and transferable methodology, such as debugging, task decomposition and abstraction, and best practices for documenting and structuring code. Targeted toward students with no programming background. May be taken for graduate credit using the LING 297 course code.
Statistical Methods in Linguists
LING 104
Fundamentals of scientific inquiry and methodology; basics of experimental design, statistical methods (descriptive, analytic, and exploratory) relevant to linguistics.
Predictive Modeling in Linguistics
LING 105
Advanced methods in quantitative linguistics. Topics may include data exploration and visualization, classification trees, random forests, regression modeling, bootstrap methods, and Bayesian data analysis. Emphasis on building predictive models of linguistic data, with a focus on simulation, model comparison, and performance evaluation. Students complete statistical programming assignments and projects using real-world data.
Foundations of Computational Linguistics
LING 110
Computational linguistics concerns the question of how computers can represent and process human language. Computational linguists develop, analyze, and apply computational models of language, both to create language technologies, and to inform the scientific understanding of language structure and usage. In this course, you will learn about, practice, and critically evaluate some of the most foundational ideas in computational linguistics.
Advanced Computational Linguistics: Text Processing
LING 111 - Spring 2021 Syllabus
Media Arts & Technology
Projects in Visualizing Information
MAT 259A
This is a ten-week comprehensive overview of visualization for Data Science, from data queries/knowledge discovery, and algorithms, resulting in projects in 2D and interactive 3D. Enrollment is limited to 15, and the course usually includes participants from Bren, COE, Geography, Statistics, Physics, Art, Political Science, etc. Students who have taken or have been teaching assistants for the course have since organized, curated paper and exhibition sessions at VISAP, Siggraph, etc. Past project results.
Molecular, Cellular, and Developmental Biology
Programming in Biology
MCDB 170
Studying complex biological systems can be greatly facilitated by modern computing technologies. This course introduces essential computer programming concepts and algorithms to biology major students. Students learn logics of programming and apply it to gene sequence analysis (bioinformatics), simulation of dynamic systems (systems biology), and data analysis (statistics in biology).
Prerequisites: MCDB 1A, EEMB 2 and MCDB 1B; and MCDB 101A or EEMB 129; and PSTAT 5A or PSTAT 5LS or MATH 4A.
Biological Dynamics
MCDB 172
An introduction to mathematical models and computer simulations used to describe and understand time varying biological systems. Learning objectives: Survey mathematical methods for describing the dependence on time of biological phenomena. Illustrate how to construct mathematical models to gain insights into complex biological systems. Develop working knowledge of a python code base that enables future evaluation of common classes of models applied to the study of biological dynamics.
Prerequisites: MCDB 1A, EEMB 2 and MCDB 1B; and MCDB 101A or EEMB 129; and either MATH 4A or MCDB 108C.
Biochemistry - Computational & Systems Biology
MCDB 108C
Models of Biochemical and Cellular Systems. Introductory systems-biology approach to model the design and the function of biological systems. Students will develop an intuition about physical concepts that are fundamental to discuss how biological organisms acquire and process information from the environment. Those concepts and tools will cover probabilities and basic dynamical systems theory. Students will build models of processes of increasing complexity, ranging from viral dynamics, bacterial resistance to drugs, the maintenance of homeostatic equilibrium (trp operon), biological oscillators (mitotic clock) and genetic switches underlying cellular decisions (bacteriophage lambda and lac operon).
Prerequisite: MCDB 108A-B; Physics 6A-B-C; Math 34A-B or Math 3A-B or Math 2A-B.
Political Science
Introduction to Research in Political Science
POLS 15
An introduction to the design and evaluation of political research, from formulating clear questions and gathering appropriate data, to the use of simple statistical techniques for analyzing data and presenting evidence.
Psychological and Brain Sciences
Statistical Methods in Psychological & Brain Sciences
PSY 10B
Introduction to key statistical concepts and their appropriate application so that students understand how to use statistics. Examines foundational statistical concepts of descriptive statistics, probability, and sampling distributions and introduces some of the major concepts and inferential statistical techniques used in psychological research to test hypotheses.
PSTAT
Understanding Data
PSTAT 5A
Introduction to data science and concepts of statistical thinking. Topics include random variables, sampling distributions, hypothesis testing, correlation and regression. Visualizing, analyzing and interpreting real world data using Python. Computing labs are required.
PSTAT 5A is not available to students in the PSTAT major. Instead, it is a common requirement for many pre-majors in the College of Letters and Science. Students interested in data science might consult with their academic advisors about the potential for replacing PSTAT 5A with the CMPSC 5A/5B sequence, which explores the subject matter in greater detail over two quarters.
Principles of Data Science with R
PSTAT 10
An overview of data analytic thinking through examples. Introduction to descriptive statistics and linear regression. Fundamentals of programming using R. Basic graphics in R. Relational database management systems and simple data manipulation using SQL.
Introduction to Statistical Machine Learning
PSTAT 131
This course explores Statistical Machine Learning to discover patterns and relationships in large data sets. Topics will include: data exploration, classification and regression tress, random forests, clustering and association rules. Building predictive models focusing on model selection, model comparison and performance evaluation. Emphasis will be on concepts, methods and data analysis using R. Students complete a significant class project, individual or team based, using real-world data.
Prerequisite: PSTAT 120A-B and PSTAT 126.
Statistical Data Science
PSTAT 134/234
Overview and use of data science tools in R and Python for data retrieval, analysis, visualization, reproducible research and automated report generation. Case studies will illustrate practical use of these tools.
Prerequisite: PSTAT 120B and PSTAT 10 and one course from Computer Science 8, or 16 or Engineering 3.
Big Data Analytics
PSTAT 135
This course introduces concepts of distributed data storage, retrieval, processing and cloud computing. Overview of methods for analyzing big data from both high dimensional statistics and machine learning - topics chosen from penalized regression, classification/clustering, dimension reduction, random projections, kernel methods, network clustering, graph analytics, supervised and unsupervised learning among others.